AI in sales enablement - going behind the hype
Everything from movie recommendations to voice assistance is being powered by Artificial Intelligence (AI). Intelligent systems are improving our lives by saving us time, reducing errors, and transforming the way we work and play. And this was before ChatGPT took the world by storm this year.
While AI is a game-changing technology, B2B technology vendors, including sales enablement platforms, are dabbling in ‘AI washing.’ This has led to customers doubting the efficacy, reliability, and promise of AI-driven products and services.
Companies are trying their best to ride the AI hype cycle. The State of AI: Divergence report found that 40% of startups that call themselves AI companies don’t use AI in a way that is ‘material’ to their business model. Most software products use rudimentary rule-based systems that don’t truly deliver on the promise and potential of intelligent automation.
Why ruled-based systems fall short
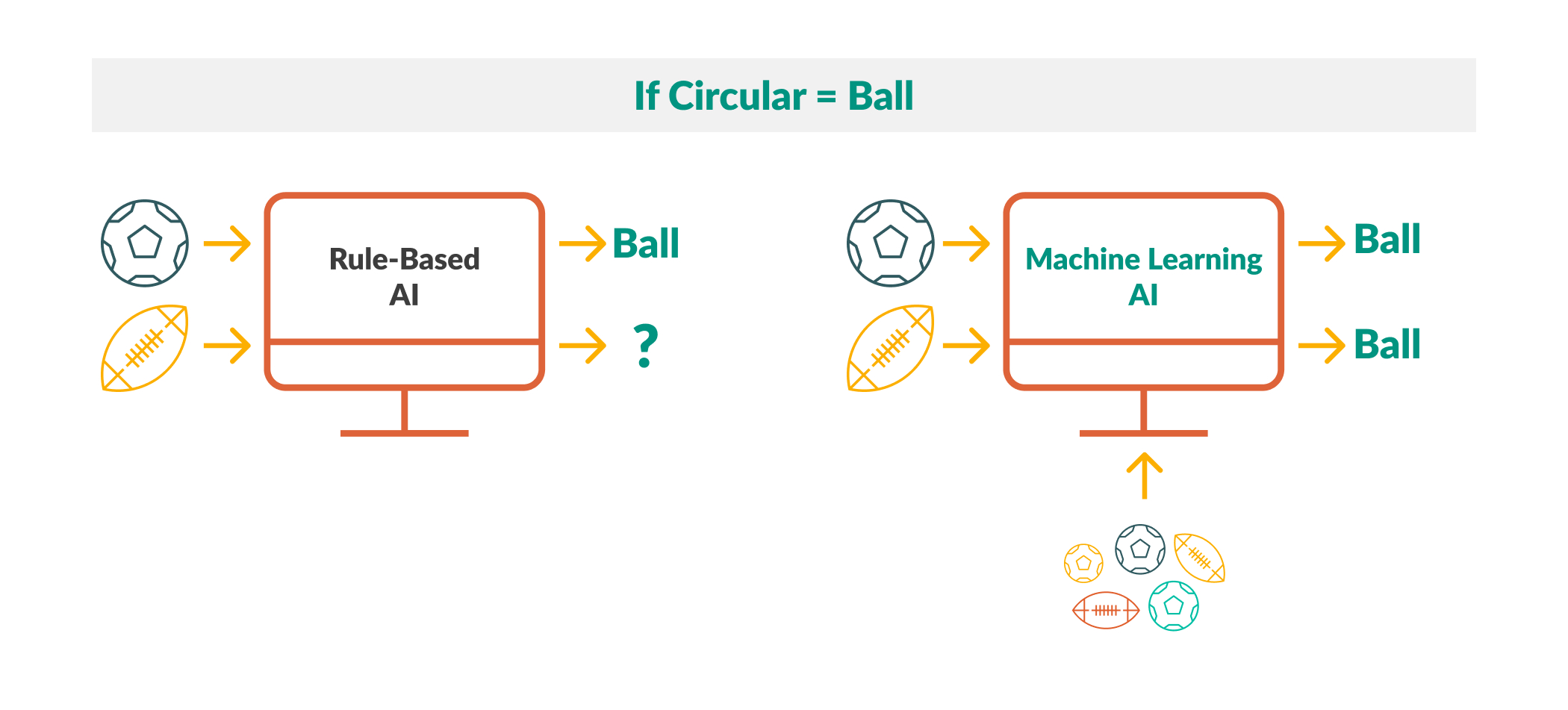
Rule-based systems are intelligent systems that use ‘if-then’ coding statements to make a decision. The two major components of rule-based models are a ‘set of rules’ and a ‘set of facts.’ However, rule-based systems have several limitations:
- It is difficult to add rules to complex business problems without introducing contradictions.
- Maintaining rule-based systems becomes difficult across complex business processes.
- Rule-based systems can’t keep up with changes in the business landscape.
Given the above context, rule-based systems are less useful for addressing problems in complex domains or across complex business processes.
Learning-based systems: Benefits and pitfalls
Learning-based AI models are a great leap forward from rule-based systems. AI-based systems learn the rules of the game on their own using training data and automatically update these rules on re-training. Learning-based systems also evolve and adapt constantly based on user engagement.
However, users can struggle with adopting AI-based products and services in their organizations. Some reasons why SaaS-based data products remain a non-essential part of the user’s workflow are inflexible training, lack of customization, incomplete context, and non-intuitive design.
Enter contextual AI for sales enablement
Contextual AI has emerged as a way to address common and recurrent problems with learning-based systems. Contextual AI systems provide intuitive and explainable results that can go a long way in building customer trust.
But, what is Contextual AI? Contextual AI takes a human approach to processing content. It is capable of analyzing the cultural, historical, and situational aspects surrounding incoming data, then using that context to determine the most meaningful outcome for the end-user.

Here are the key pillars of Contextual AI that deliver just-in-time sales enablement:
- Intelligible. Most predictions from AI systems are black boxes. It is hard for users to trust the results without understanding the reasons for a recommendation. Results should be intuitive and explainable: ‘we recommend product A as it is frequently bought with product B.’
- Adaptive. Contextual AI models are intelligent enough to adapt their recommendations based on the use case and business intention. For example, in the travel domain, hotel recommendations for family vacations and business travel are quite different and a recommendation system needs to adapt to the same.
- Customizable. AI systems need extensive training before they can recognize data patterns. Unfortunately, training datasets are far removed from the user’s actual problem domain which can result in sub-optimal performance across production scenarios. While no model can be trained with unlimited data, Contextual AI systems can add custom knowledge to address different use cases. Adaptability and customization increase user trust and confidence with AI tools.
- Context-Aware. Most business problems are multi-layered and require the system to factor in different behavior based on context. Contextual AI systems are deeply integrated with human-level context and offer a 360-degree view of the problem.
Just-in-time enablement requires contextual AI
Sales enablement AI platforms such as GTM Buddy’s Contextual AI can learn the overall business context without frequent retraining while also taking relevant inputs from customers.
Recommendations are powerful when they are contextual, intuitive, and tailored to the seller’s workflow. At GTM Buddy, we are bringing in multilayered intelligence using multidimensional context so that sellers can share relevant and useful content with their prospects.
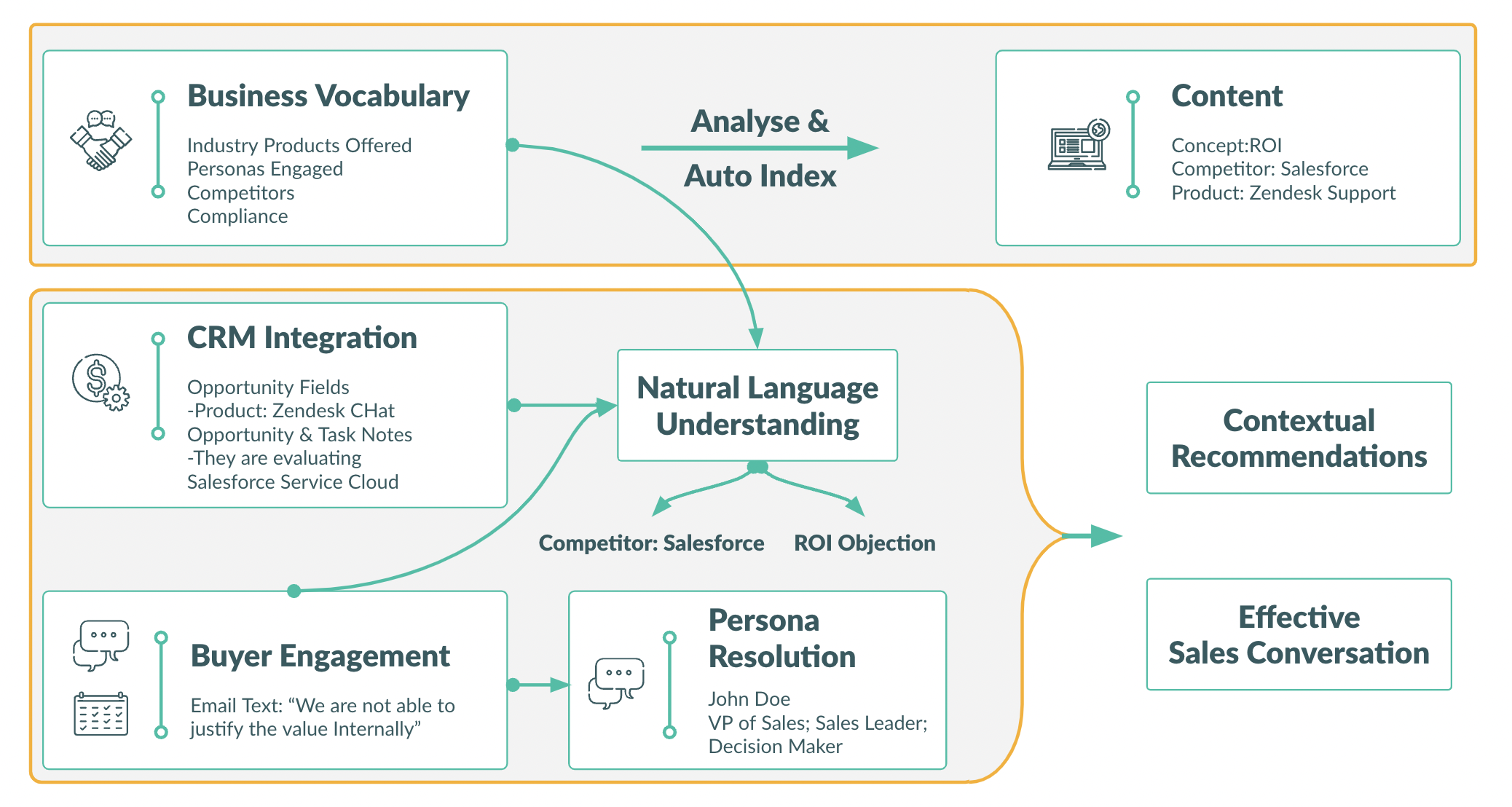
Gain the right buyer context
The bedrock of GTM Buddy’s intelligence layer is 360-degree buyer context. GTM Buddy’s sales enablement AI platform considers the overall business, opportunity, engagement, persona, and content context before making any recommendation.
GTM Buddy delivers relevant and just-in-time information for sellers with:
CRM Integration. CRM is a first-class citizen in GTM Buddy. CRM tools offer context on the opportunity, business landscape, buyer engagement, conversations, and previous deal history. Deep CRM integration enables GTM Buddy’s intelligence layer to understand the opportunity, account, contact, and buyer personas so that it can surface the right information at the right time for sellers.
Business Vocabulary. All revenue teams sell specific products and services, handle objections on price, return on investment, and time to value, offer customer evidence, ensure vertical-specific customization, and deal with competitors. While each deal might have common structural elements, the details of an opportunity are specific to each buyer. AI models for sales have to understand these common components while adapting to the specific context of a customer.
GTM Buddy’s Deep Learning language models are adaptable and customizable. These models can optimize recommendations based on the business vocabulary provided by a seller. Check out this blog on business vocabulary for more details.
Buyer Engagement, Conversations, and Deal History. GTM Buddy uses Bayesian probabilistic models to understand buyer engagement and deal history. The intelligence layer connects each contact to a buyer persona and measures content engagement by persona and deal stage. It also knows when a piece of content is relevant to a specific buyer, tracks the average time for closing deals, and measures which kinds of content have an impact on deal closures.
Intuitive and Explainable. GTM Buddy uses the tools and frameworks of Explainable AI to provide intuitive seller recommendations. Sellers can easily understand that a recommended case study is relevant as it explains the ROI delivered for a specific persona and against a particular competitor.
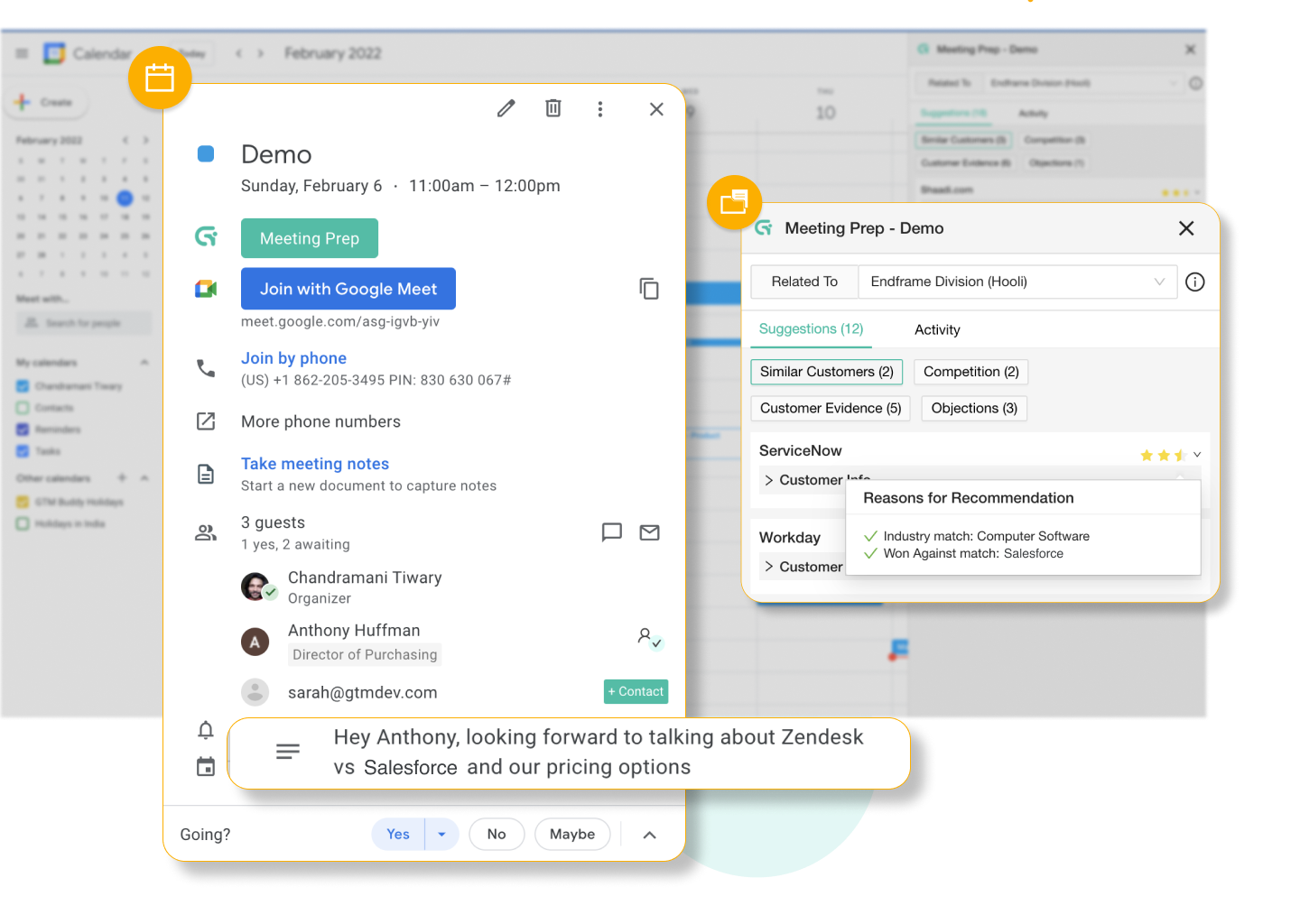
How natural language understanding delivers semantic context

GTM Buddy uses Natural Language Understanding (NLU) techniques based on Deep Learning to understand the intent and entities of interest for users.
Intent. In a sales context, intent revolves around the type of content, the intent could be to share customer evidence, find competitive insights, and so on. AI systems should be able to understand whether a buyer is requesting customer evidence or if the competitive battle card is relevant for a specific opportunity.
GTM Buddy has created unique text classification models to predict the type of content request. When a buyer asks for case studies in manufacturing, the system classifies this as a request for customer evidence.
Entities. Sales teams deal with common entities such as products, competitors, industries, and geographies. GTM Buddy has built a named entity recognition model to classify intent as well as identify relevant entities.
A case study in the manufacturing industry is identified as an industry entity by GTM Buddy. The capability to classify intent and identify entities helps our Intelligence layer answer the following questions:
- What information can be found in this content?
- What are the concerns expressed by a buyer?
- What information is the seller looking for?
Understanding sales concepts is critical
GTM Buddy’s language models are trained to understand important sales concepts. For example, if a buyer is struggling to justify the value of your product to their internal teams, GTM Buddy links this issue to the sales concept of ROI.
Why smart similarities matter
GTM Buddy’s sales enablement AI platform makes recommendations based on an understanding of similarities. Providing relevant customer evidence is critical to winning a deal. GTM Buddy connects the context of a current opportunity with existing customers and then delivers relevant case study recommendations for sellers.
The notion of similarity is baked into our Intelligence layer. We support the following types of similarities:
- Sentence – Sentence similarity is used as an input in the content recommendation engine. Our language model enables us to score similarities between buyer questions and information available in the content hub.
- Customer – GTM Buddy can measure the resemblance between a current opportunity and existing customers by combining different similarities such as:
• Segment Similarity. Is a customer who purchased 1,000 seats more similar to a customer that bought 1,250 seats? Or, one that purchased 750 seats?
• Industry Similarity. Is a customer from a life sciences organization referenceable to a healthcare opportunity?
• Region Similarity. Is an APAC customer referenceable for an opportunity in New Zealand? - Job Title – Job titles help GTM Buddy’s intelligence layer map relevant contacts to the right personas and recommend useful content for each persona.
Summary
Sellers have to constantly re-adjust to different buying signals while working on an opportunity. Products designed for sellers need to adapt to a rep’s daily workflow, gain context by understanding different buyer interactions, and offer relevant content recommendations to drive effective sales conversations. GTM Buddy’s just-in-time enablement solution uses Contextual AI so that reps can access all the information they need to sell better without having to leave the tools (email, calendar, and CRM) that are part of their workflow.
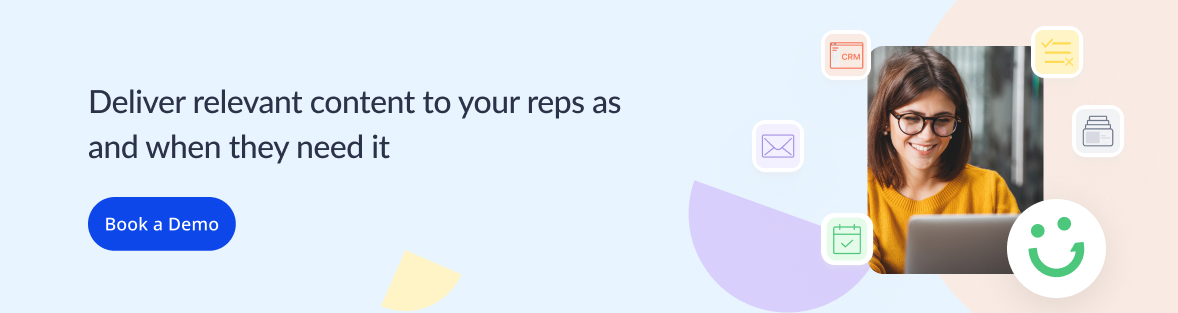